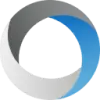
Radicalbit
The MLOps & AI Observability Platform for Real-Time AI Applications
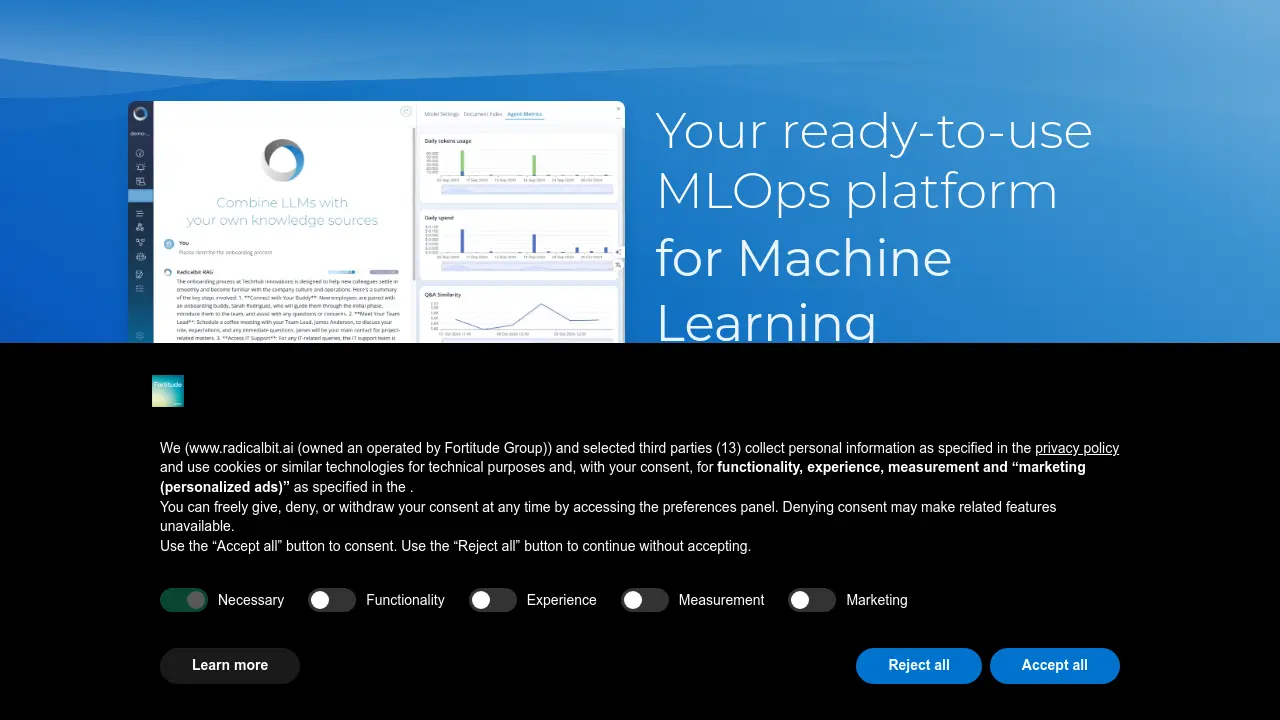
Description
Radicalbit provides a comprehensive MLOps and AI Observability platform engineered to enhance the deployment, serving, monitoring, and explanation of various AI models, including Machine Learning, Computer Vision, and Large Language Models (LLMs). It empowers data teams to maintain complete control over the entire data lifecycle through capabilities like real-time data exploration, detection of outliers and drift, and continuous model monitoring while in production.
The platform aims to significantly reduce the time-to-value for AI applications, offering substantial cost savings through automation and proactive issue identification like outlier and drift detection. It ensures scalability with features like scale-to-zero and automated resource management, while also supporting governance and compliance efforts through advanced monitoring, observability, and model explainability features designed to foster fairness and transparency, aligning with regulations such as the European Union AI Act. Radicalbit integrates seamlessly into existing ML stacks and can be deployed either as SaaS or on-premise.
Key Features
- AI Model Deployment & Serving: Deploy MLflow or Hugging Face models for ML, Computer Vision, and LLMs via UI or APIs.
- Real-Time Data Transformation: Design and run data pipelines using a visual canvas or custom Python code.
- Data Integrity Monitoring: Mitigate data/concept drift, identify missing values/outliers, and manage schema evolution.
- AI Observability: Track model activity and performance in real-time, with auto-retraining triggers for continual learning.
- Model Explainability: Understand AI model outputs to avoid bias, ensure compliance, and optimize processes.
- LLM Evaluation: Assess the performance of Large Language Models.
- RAG Application Management: Develop and monitor custom Retrieval-Augmented Generation applications.
- Built-in Feature Store: Securely store online and offline features and predictions.
- Flexible Deployment: Available as SaaS or on-premise on private cloud or own infrastructure.
- Automated Resource Management: Adjust workloads and save energy with scale-to-zero capabilities.
Use Cases
- Streamlining the MLOps lifecycle for AI applications.
- Deploying and serving ML, Computer Vision, and LLM models at scale.
- Monitoring AI models in production for performance degradation and drift.
- Ensuring data integrity and quality for AI systems.
- Explaining AI model behavior for compliance and optimization.
- Developing and monitoring Retrieval-Augmented Generation (RAG) applications.
- Reducing time-to-value for AI projects.
- Achieving AI governance and regulatory compliance (e.g., EU AI Act).
You Might Also Like
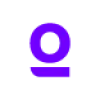
Genius Offer AI
FreemiumMake Offers So Good People Will Feel Stupid Saying No
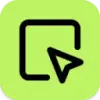
Makeweb.ai
PaidChat with AI to Make your Websites
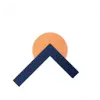
Heydai
OtherDaily planner & time tracker
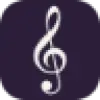
MUSICTOMIDI
FreeConvert Music to MIDI Online Using Advanced AI Technology
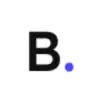
BlogPilot
Free TrialBoost Your SEO with Automated Content Clusters